사이킷런 튜토리얼#
Attention
빅분기 문제 해결을 위한 사이킷런 모듈 정리
url =https://scikit-learn.org/stable/modules/classes.html#module
모듈이용
from import를 이용하여 사용하고자 하는 모듈만 import 합니다.
from sklearn.preprocessing import MinMaxScaler
sc = MinMaxScaler()
세부 카테고리 검색
중간가지의 모듈들 정도는 암기하시고 dir 함수를 이용하여 세부 카테고리는 검색해가며 사용하면 좋습니다.
import sklearn.model_selection
dir(sklearn.model_selection)[:5]
['BaseCrossValidator',
'GridSearchCV',
'GroupKFold',
'GroupShuffleSplit',
'KFold']
all 을 이용한 탐색
import sklearn 만 진행한후 __all__을 이용해서 하위 모듈들을 확인할 수 있습니다.
import sklearn
sklearn.__all__[-15:-10]
['neural_network',
'pipeline',
'preprocessing',
'random_projection',
'semi_supervised']
import sklearn.preprocessing
sklearn.preprocessing.__all__[:6]
['Binarizer',
'FunctionTransformer',
'KBinsDiscretizer',
'KernelCenterer',
'LabelBinarizer',
'LabelEncoder']
모듈리스트#
sklearn
│
├── 01 preprocessing (전처리)
│ │
│ ├── 스케일러
│ │ ├── MinMaxScaler
│ │ ├── RobustScaler
│ │ └── StandardScaler
│ │
│ └── 인코더
│ ├── LabelEncoder
│ └── OneHotEncoder
│
├── 02 model_selection (모델링 전처리)
│ │
│ ├── 데이터셋 분리
│ │ ├── KFold
│ │ ├── StratifiedKFold
│ │ └── train_test_split
│ │
│ └── 하이퍼파라미터 튜닝
│ └── GridSearchCV
│
├── 03 모델학습
│ │
│ ├── ensemble
│ │ ├── AdaBoostClassifier
│ │ ├── GradientBoostingClassifier
│ │ ├── RandomForestClassifier
│ │ └── RandomForestRegressor
│ │
│ ├── linear_model
│ │ ├── LogisticRegression
│ │ └── RidgeClassifier
│ │
│ ├── neighbors
│ │ └── KNeighborsClassifier
│ │
│ ├── svm
│ │ ├── SVC
│ │ └── SVR
│ │
│ └── tree
│ ├── DecisionTreeClassifier
│ ├── DecisionTreeRegressor
│ ├── ExtraTreeClassifier
│ └── ExtraTreeRegressor
│
├── 04 모델평가
│ │
│ ├── metrics
│ │ ├── accuracy_score
│ │ ├── classification_report
│ │ ├── confusion_matrix
│ │ ├── f1_score
│ │ ├── log_loss
│ │ ├── mean_absolute_error
│ │ ├── mean_squared_error
│ │ └── roc_auc_score
│ │
│ └── model (정의된 모델에서 추출)
│ ├── predict
│ └── predict_proba
│
└── 05 최종앙상블
│
└── ensemble
├── StackingClassifier
├── StackingRegressor
├── VotingClassifier
└── VotingRegressor
01 데이터 전처리#
MinMaxScaler#
Attention
데이터 설명 : 비행탑승 경험 만족도
x_train: https://raw.githubusercontent.com/Datamanim/datarepo/main/airline/x_train.csv
x_test: https://raw.githubusercontent.com/Datamanim/datarepo/main/airline/x_test.csv
출처 (참고, 데이터 수정)
import pandas as pd
train = pd.read_csv('https://raw.githubusercontent.com/Datamanim/datarepo/main/airline/x_train.csv')
test = pd.read_csv('https://raw.githubusercontent.com/Datamanim/datarepo/main/airline/x_test.csv')
display(train.head(2))
ID | Gender | Customer Type | Age | Type of Travel | Class | Flight Distance | Inflight wifi service | Departure/Arrival time convenient | Ease of Online booking | ... | Inflight entertainment | On-board service | Leg room service | Baggage handling | Checkin service | Inflight service | Cleanliness | Departure Delay in Minutes | Arrival Delay in Minutes | id | |
---|---|---|---|---|---|---|---|---|---|---|---|---|---|---|---|---|---|---|---|---|---|
0 | 0 | Female | Loyal Customer | 54 | Personal Travel | Eco | 1068 | 3 | 4 | 3 | ... | 5 | 5 | 3 | 5 | 3 | 5 | 3 | 47 | 22.0 | NaN |
1 | 2 | Male | Loyal Customer | 20 | Personal Travel | Eco | 1546 | 4 | 4 | 4 | ... | 4 | 3 | 3 | 4 | 4 | 4 | 4 | 5 | 2.0 | NaN |
2 rows × 24 columns
Question 1
train 데이터의 Flight Distance 컬럼을 사이킷런 모듈을 이용하여 최솟값을 0 최댓값을 1값로 하는 데이터로 변환하고 scaling을 이름으로 하는 컬럼으로 데이터프레임에 추가하라
Show code cell source
from sklearn.preprocessing import MinMaxScaler
mm = MinMaxScaler()
mm.fit(train['Flight Distance'].values.reshape(-1,1))
scalingdata = mm.transform(train['Flight Distance'].values.reshape(-1,1))
train['scaling'] = scalingdata
##결과 시각화
import matplotlib.pyplot as plt
fig , ax = plt.subplots(1,2)
ax[0].boxplot(train['Flight Distance'])
ax[0].set_xticks([1])
ax[0].set_xticklabels(['Raw'])
ax[1].boxplot(scalingdata)
ax[1].set_xticks([1])
ax[1].set_xticklabels(['Scaling data'])
plt.show()
# 분포는 바뀌지 않는다
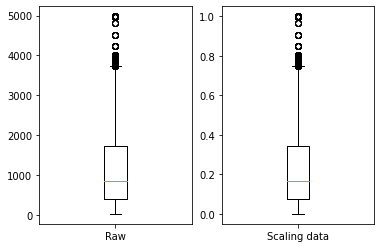
Question 2
train 데이터의 Flight Distance 컬럼을 pandas의 내장함수만을 이용하여 최솟값을 0 최댓값을 1값로 하는 데이터로 변환하고 scaling을 이름으로 하는 컬럼으로 데이터프레임에 추가하라
Show code cell source
scaling = (train['Flight Distance'] - train['Flight Distance'].min()) /(train['Flight Distance'].max() - train['Flight Distance'].min())
train['scaling'] = scaling
##결과 시각화
import matplotlib.pyplot as plt
fig , ax = plt.subplots(1,2)
ax[0].boxplot(train['Flight Distance'])
ax[0].set_xticks([1])
ax[0].set_xticklabels(['Raw'])
ax[1].boxplot(scalingdata)
ax[1].set_xticks([1])
ax[1].set_xticklabels(['Scaling data'])
plt.show()
# 분포는 바뀌지 않는다
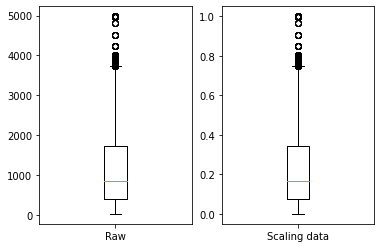
Question 3
train 데이터의 Age컬럼을 MinMax 스케일링 진행 하고 age_scaling컬럼에 추가하고 train셋과 같은 기준으로 test데이터의 Age를 스케일링하여 age_scaling에 추가하라
Show code cell source
from sklearn.preprocessing import MinMaxScaler
mm = MinMaxScaler()
mm.fit(train['Age'].values.reshape(-1,1))
train['age_scaling'] = mm.transform(train['Age'].values.reshape(-1,1))
test['age_scaling'] = mm.transform(test['Age'].values.reshape(-1,1))
display(test[['ID','age_scaling']].head(3))
## 짧게 쓴다면 아래와 같이 쓸수도 있다
# mm = MinMaxScaler()
# train['age_scaling'] = mm.fit_transform(train['Age'].values.reshape(-1,1))
# test['age_scaling'] = mm.transform(test['Age'].values.reshape(-1,1))
ID | age_scaling | |
---|---|---|
0 | 1 | 0.269231 |
1 | 16 | 0.346154 |
2 | 17 | 0.205128 |
StandardScaler#
Question 4
train 데이터의 Age컬럼을 pandas 기본 내장 모듈을 이용하여 정규화 스케일링을 진행 하고 age_scaling컬럼에 추가하라
Show code cell source
scaling_ddof1 = (train['Age'] - train['Age'].mean()) /(train['Age'].std())
train['scaling'] = scaling_ddof1
scaling_ddof0 = (train['Age'] - train['Age'].mean()) /(train['Age'].std(ddof=0))
train['scaling_ddof0'] = scaling_ddof0
##결과 시각화
import matplotlib.pyplot as plt
fig , ax = plt.subplots(1,2)
ax[0].boxplot(train['scaling'])
ax[0].set_xticks([1])
ax[0].set_xticklabels(['ddof 0 \ndefault'])
ax[1].boxplot(train['scaling_ddof0'])
ax[1].set_xticks([1])
ax[1].set_xticklabels(['ddof 1'])
plt.show()
from IPython.display import display_html
df1 = train[['scaling']].describe()
df2 = train[['scaling_ddof0']].describe()
df1_styler = df1.style.set_table_attributes("style='display:inline'").set_caption('Caption table 1')
df2_styler = df2.style.set_table_attributes("style='display:inline'").set_caption('Caption table 2')
display_html(df1_styler._repr_html_()+df2_styler._repr_html_(), raw=True)
# ddof에 따라 큰 차이가 없다
# 실제로 ddof의 문서에서도 모델 학습에 있어서 큰차이 가 없다는 표현이 있음
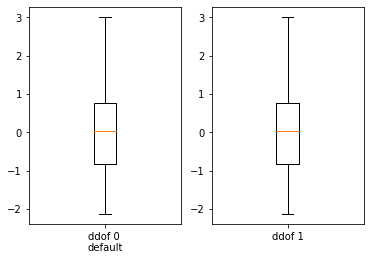
scaling | |
---|---|
count | 83123.000000 |
mean | 0.000000 |
std | 1.000000 |
min | -2.142709 |
25% | -0.818424 |
50% | 0.042361 |
75% | 0.770717 |
max | 3.022001 |
scaling_ddof0 | |
---|---|
count | 83123.000000 |
mean | 0.000000 |
std | 1.000006 |
min | -2.142722 |
25% | -0.818429 |
50% | 0.042361 |
75% | 0.770722 |
max | 3.022019 |
Question 5
train 데이터의 Age컬럼을 sklearn 모듈을 이용하여 정규화 스케일링을 진행 하고 age_scaling컬럼에 추가하고 train셋과 같은 기준으로 test데이터의 Age를 스케일링하여 age_scaling에 추가하라
Show code cell source
from sklearn.preprocessing import StandardScaler
sc = StandardScaler()
sc.fit(train['Age'].values.reshape(-1,1))
train['age_scaling'] = sc.transform(train['Age'].values.reshape(-1,1))
test['age_scaling'] = sc.transform(test['Age'].values.reshape(-1,1))
display(test[['ID','age_scaling']].head(3))
## 짧게 쓴다면 아래와 같이 쓸수도 있다
# sc = StandardScaler()
# train['age_scaling'] = sc.fit_transform(train['Age'].values.reshape(-1,1))
# test['age_scaling'] = sc.transform(test['Age'].values.reshape(-1,1))
##결과 시각화
import matplotlib.pyplot as plt
fig , ax = plt.subplots(1,2)
ax[0].boxplot(train['Age'])
ax[0].set_xticks([1])
ax[0].set_xticklabels(['Raw'])
ax[1].boxplot(train['age_scaling'])
ax[1].set_xticks([1])
ax[1].set_xticklabels(['age_scaling'])
plt.show()
ID | age_scaling | |
---|---|---|
0 | 1 | -0.752215 |
1 | 16 | -0.354927 |
2 | 17 | -1.083288 |
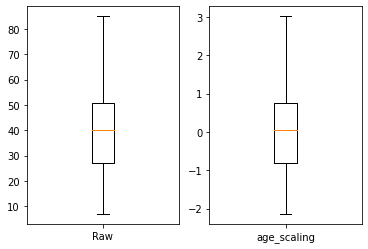
LabelEnconder#
Attention
데이터 설명 : 이직여부 판단 데이터
train: https://raw.githubusercontent.com/Datamanim/datarepo/main/HRdata/X_train.csv
test : https://raw.githubusercontent.com/Datamanim/datarepo/main/HRdata/y_train.csv
출처 (참고, 데이터 수정)
import pandas as pd
#데이터 로드
train = pd.read_csv("https://raw.githubusercontent.com/Datamanim/datarepo/main/HRdata/X_train.csv")
test= pd.read_csv("https://raw.githubusercontent.com/Datamanim/datarepo/main/HRdata/X_test.csv")
display(train.head(2))
enrollee_id | city | city_development_index | gender | relevent_experience | enrolled_university | education_level | major_discipline | experience | company_size | company_type | last_new_job | training_hours | |
---|---|---|---|---|---|---|---|---|---|---|---|---|---|
0 | 25298 | city_138 | 0.836 | Male | No relevent experience | Full time course | High School | NaN | 5 | 100-500 | Pvt Ltd | 1 | 45 |
1 | 4241 | city_160 | 0.920 | Male | No relevent experience | Full time course | High School | NaN | 5 | NaN | NaN | 1 | 17 |
OneHotEncoder#
multi-columns에 대해서 작업가능한 pandas의 pd.get_dummies가 더 좋음!
02 모델링 전처리#
train_test_split#
Attention
데이터 설명 : 투약하는 약을 분류 (종속변수 :Drug) x_train: https://raw.githubusercontent.com/Datamanim/datarepo/main/drug/x_train.csv y_train: https://raw.githubusercontent.com/Datamanim/datarepo/main/drug/y_train.csv 출처
import pandas as pd
x = pd.read_csv("https://raw.githubusercontent.com/Datamanim/datarepo/main/drug/x_train.csv")
y = pd.read_csv("https://raw.githubusercontent.com/Datamanim/datarepo/main/drug/y_train.csv")[['Drug']]
display(x.head(2))
display(y.head(2))
ID | Age | Sex | BP | Cholesterol | Na_to_K | |
---|---|---|---|---|---|---|
0 | 0 | 36 | F | NORMAL | HIGH | 16.753 |
1 | 1 | 47 | F | LOW | HIGH | 11.767 |
Drug | |
---|---|
0 | 0 |
1 | 3 |
Question 6
x,y데이터에서 train,test세트를 구분하고 train셋의 y값과 test셋의 y값의 unique한 value 값의 숫자를 출력하라. train:test는 7:3비율 , random_state =42로 고정
Show code cell source
from sklearn.model_selection import train_test_split
X_train, X_test ,y_train,y_test =train_test_split(x,y,test_size=0.3,random_state =42)
y.columns = ['Class']
t = pd.concat([y_train.value_counts(),y_test.value_counts()],axis=1).rename(columns={0:'train',1:'test'}).reset_index()
t
# train, test에서 Class별 비율이 다른것을 확인 할 수 있다
Class | train | test | |
---|---|---|---|
0 | 0 | 54 | 18 |
1 | 1 | 13 | 5 |
2 | 2 | 8 | 4 |
3 | 3 | 5 | 7 |
4 | 4 | 29 | 14 |
Question 7
x,y데이터에서 train,test세트를 구분하고 train셋의 y값과 test셋의 y값의 unique한 value 값의 비율을 동일하게 추출하라. 7:3비율 , random_state =42로 고정
Show code cell source
from sklearn.model_selection import train_test_split
X_train, X_test ,y_train,y_test =train_test_split(x,y,test_size=0.3,random_state =42,stratify=y)
y.columns = ['Class']
t = pd.concat([y_train.value_counts(),y_test.value_counts()],axis=1).rename(columns={0:'train',1:'test'}).reset_index()
display(t)
# 비율 동일
Class | train | test | |
---|---|---|---|
0 | 0 | 50 | 22 |
1 | 4 | 30 | 13 |
2 | 1 | 13 | 5 |
3 | 3 | 8 | 4 |
4 | 2 | 8 | 4 |
03 모델링#
모델링의 기본 골격
model import
model 선언, 초기 하이퍼 파라미터 지정
model.fit(x_train,y_train)을 통한 모델 학습
회귀, 분류 문제 모두 model.predict(x_validation) 을 통한 예측
auc값을 구해야하는 경우 model.predict_proba(x_validation)을 통한 확률 추출 (svm모델의 경우 학습시 probability=True옵션 추가)
원하는 metric으로 모델 평가 eg) accuracy_score(y_validation, model.predict(x_validation))
Tip
어떤 모델을 써야할지 잘모르겠다 싶으면 랜덤포레스트쓰면 중간이상은 한다!
RandomForestClassifier , RandomForestRegressor
Tip
모듈 경로를 못외울때는 아래와 같은 방법접근
sklearn 하위 첫번째 모듈까지는 외워야만 한다.
import sklearn.ensemble
dir(sklearn.ensemble)[:10]
['AdaBoostClassifier',
'AdaBoostRegressor',
'BaggingClassifier',
'BaggingRegressor',
'BaseEnsemble',
'ExtraTreesClassifier',
'ExtraTreesRegressor',
'GradientBoostingClassifier',
'GradientBoostingRegressor',
'IsolationForest']
sklearn 학습 모듈 import 모음
분류문제의 경우 -Classifier , 회귀문제의 경우 -Regressor 형식을 가진다.
사이킷런 모듈 버전따라 조금씩 다를수 있음
#ensemble
from sklearn.ensemble import AdaBoostClassifier
from sklearn.ensemble import AdaBoostRegressor
from sklearn.ensemble import BaggingClassifier
from sklearn.ensemble import BaggingRegressor
from sklearn.ensemble import ExtraTreesClassifier
from sklearn.ensemble import ExtraTreesRegressor
from sklearn.ensemble import GradientBoostingClassifier
from sklearn.ensemble import GradientBoostingRegressor
from sklearn.ensemble import RandomForestClassifier
from sklearn.ensemble import RandomForestRegressor
#linear_model
from sklearn.linear_model import BayesianRidge
from sklearn.linear_model import ElasticNet
from sklearn.linear_model import GammaRegressor
from sklearn.linear_model import HuberRegressor
from sklearn.linear_model import Lasso
from sklearn.linear_model import LinearRegression
from sklearn.linear_model import LogisticRegression # 분류
from sklearn.linear_model import Ridge
from sklearn.linear_model import SGDRegressor
from sklearn.linear_model import SGDClassifier # 분류
#neighbors
from sklearn.neighbors import KNeighborsClassifier
from sklearn.neighbors import KNeighborsRegressor
from sklearn.neighbors import KNeighborsTransformer
from sklearn.neighbors import NearestNeighbors
#svm
from sklearn.svm import LinearSVC
from sklearn.svm import LinearSVR
from sklearn.svm import OneClassSVM
from sklearn.svm import SVR # regression
from sklearn.svm import SVC # classfier
#tree
from sklearn.tree import BaseDecisionTree
from sklearn.tree import DecisionTreeClassifier
from sklearn.tree import DecisionTreeRegressor
from sklearn.tree import ExtraTreeClassifier
from sklearn.tree import ExtraTreeRegressor
04 모델 평가#
import sklearn.metrics
dir(sklearn.metrics)
['ConfusionMatrixDisplay',
'DetCurveDisplay',
'PrecisionRecallDisplay',
'RocCurveDisplay',
'SCORERS',
'__all__',
'__builtins__',
'__cached__',
'__doc__',
'__file__',
'__loader__',
'__name__',
'__package__',
'__path__',
'__spec__',
'_base',
'_classification',
'_pairwise_fast',
'_plot',
'_ranking',
'_regression',
'_scorer',
'accuracy_score',
'adjusted_mutual_info_score',
'adjusted_rand_score',
'auc',
'average_precision_score',
'balanced_accuracy_score',
'brier_score_loss',
'calinski_harabasz_score',
'check_scoring',
'classification_report',
'cluster',
'cohen_kappa_score',
'completeness_score',
'confusion_matrix',
'consensus_score',
'coverage_error',
'davies_bouldin_score',
'dcg_score',
'det_curve',
'euclidean_distances',
'explained_variance_score',
'f1_score',
'fbeta_score',
'fowlkes_mallows_score',
'get_scorer',
'hamming_loss',
'hinge_loss',
'homogeneity_completeness_v_measure',
'homogeneity_score',
'jaccard_score',
'label_ranking_average_precision_score',
'label_ranking_loss',
'log_loss',
'make_scorer',
'matthews_corrcoef',
'max_error',
'mean_absolute_error',
'mean_absolute_percentage_error',
'mean_gamma_deviance',
'mean_poisson_deviance',
'mean_squared_error',
'mean_squared_log_error',
'mean_tweedie_deviance',
'median_absolute_error',
'multilabel_confusion_matrix',
'mutual_info_score',
'nan_euclidean_distances',
'ndcg_score',
'normalized_mutual_info_score',
'pair_confusion_matrix',
'pairwise',
'pairwise_distances',
'pairwise_distances_argmin',
'pairwise_distances_argmin_min',
'pairwise_distances_chunked',
'pairwise_kernels',
'plot_confusion_matrix',
'plot_det_curve',
'plot_precision_recall_curve',
'plot_roc_curve',
'precision_recall_curve',
'precision_recall_fscore_support',
'precision_score',
'r2_score',
'rand_score',
'recall_score',
'roc_auc_score',
'roc_curve',
'silhouette_samples',
'silhouette_score',
'top_k_accuracy_score',
'v_measure_score',
'zero_one_loss']
05 최종 앙상블#
조만간..