17ν
17ν#
Attention
μΊκΈμ μ
λ‘λλ λ€λ₯Έ λΆλ€ μ½λ 보λ¬κ°κΈ°
λ°μ΄ν°μ
λ§ν¬
λ¬Έμ μ€λ₯, μ½λμ€λ₯ λκΈλ‘ νΌλλ°±μ£ΌμΈμ
Attention
1λ²
λ°μ΄ν° μ€λͺ
: μ§κ³Ό κ΄λ ¨λ μ¬λ¬ μμΉλ€κ³Ό μ§μ κ°κ²©, log1p μ κ·νλ price μ»¬λΌ μμΈ‘ νκΈ°
λ°μ΄ν° μΆμ² : https://www.kaggle.com/c/house-prices-advanced-regression-techniques/data?select=train.csv μΌλΆ μ μ²λ¦¬
data Url : https://raw.githubusercontent.com/Datamanim/datarepo/main/adp/p3/problem1.csv
import pandas as pd
df = pd.read_csv('https://raw.githubusercontent.com/Datamanim/datarepo/main/adp/p3/problem1.csv')
df.head()
Id | LotArea | LotFrontage | YearBuilt | 1stFlrSF | 2ndFlrSF | YearRemodAdd | TotRmsAbvGrd | KitchenAbvGr | BedroomAbvGr | GarageCars | GarageArea | price | |
---|---|---|---|---|---|---|---|---|---|---|---|---|---|
0 | 1 | 8450 | 65.0 | 2003 | 856 | 854 | 2003 | 8 | 1 | 3 | 2 | 548 | 12.247699 |
1 | 2 | 9600 | 80.0 | 1976 | 1262 | 0 | 1976 | 6 | 1 | 3 | 2 | 460 | 12.109016 |
2 | 3 | 11250 | 68.0 | 2001 | 920 | 866 | 2002 | 6 | 1 | 3 | 2 | 608 | 12.317171 |
3 | 4 | 9550 | 60.0 | 1915 | 961 | 756 | 1970 | 7 | 1 | 3 | 3 | 642 | 11.849405 |
4 | 5 | 14260 | 84.0 | 2000 | 1145 | 1053 | 2000 | 9 | 1 | 4 | 3 | 836 | 12.429220 |
1-1λ²
λ°μ΄ν° EDA μν ν, λΆμκ° μ μ₯μμ μλ―Έμλ νμ
μκ°ν λ° ν΅κ³λ μ μ
print(df.info())
display(df.describe())
print('''
λͺ¨λ 컬λΌμ numeric λ³μμ΄λ€. μ΄μμΉκ° μ‘΄μ¬νλ 컬λΌμ ~~ μ΄λ€. (μ€λ΅)
''')
import matplotlib.pyplot as plt
df.plot(kind='box',subplots=True,layout=(2,len(df.columns)//2+1),figsize=(20,10))
plt.tight_layout()
plt.show()
<class 'pandas.core.frame.DataFrame'>
RangeIndex: 1460 entries, 0 to 1459
Data columns (total 13 columns):
# Column Non-Null Count Dtype
--- ------ -------------- -----
0 Id 1460 non-null int64
1 LotArea 1460 non-null int64
2 LotFrontage 1201 non-null float64
3 YearBuilt 1460 non-null int64
4 1stFlrSF 1460 non-null int64
5 2ndFlrSF 1460 non-null int64
6 YearRemodAdd 1460 non-null int64
7 TotRmsAbvGrd 1460 non-null int64
8 KitchenAbvGr 1460 non-null int64
9 BedroomAbvGr 1460 non-null int64
10 GarageCars 1460 non-null int64
11 GarageArea 1460 non-null int64
12 price 1460 non-null float64
dtypes: float64(2), int64(11)
memory usage: 148.4 KB
None
Id | LotArea | LotFrontage | YearBuilt | 1stFlrSF | 2ndFlrSF | YearRemodAdd | TotRmsAbvGrd | KitchenAbvGr | BedroomAbvGr | GarageCars | GarageArea | price | |
---|---|---|---|---|---|---|---|---|---|---|---|---|---|
count | 1460.000000 | 1460.000000 | 1201.000000 | 1460.000000 | 1460.000000 | 1460.000000 | 1460.000000 | 1460.000000 | 1460.000000 | 1460.000000 | 1460.000000 | 1460.000000 | 1460.000000 |
mean | 730.500000 | 10516.828082 | 70.049958 | 1971.267808 | 1162.626712 | 346.992466 | 1984.865753 | 6.517808 | 1.046575 | 2.866438 | 1.767123 | 472.980137 | 12.024057 |
std | 421.610009 | 9981.264932 | 24.284752 | 30.202904 | 386.587738 | 436.528436 | 20.645407 | 1.625393 | 0.220338 | 0.815778 | 0.747315 | 213.804841 | 0.399449 |
min | 1.000000 | 1300.000000 | 21.000000 | 1872.000000 | 334.000000 | 0.000000 | 1950.000000 | 2.000000 | 0.000000 | 0.000000 | 0.000000 | 0.000000 | 10.460271 |
25% | 365.750000 | 7553.500000 | 59.000000 | 1954.000000 | 882.000000 | 0.000000 | 1967.000000 | 5.000000 | 1.000000 | 2.000000 | 1.000000 | 334.500000 | 11.775105 |
50% | 730.500000 | 9478.500000 | 69.000000 | 1973.000000 | 1087.000000 | 0.000000 | 1994.000000 | 6.000000 | 1.000000 | 3.000000 | 2.000000 | 480.000000 | 12.001512 |
75% | 1095.250000 | 11601.500000 | 80.000000 | 2000.000000 | 1391.250000 | 728.000000 | 2004.000000 | 7.000000 | 1.000000 | 3.000000 | 2.000000 | 576.000000 | 12.273736 |
max | 1460.000000 | 215245.000000 | 313.000000 | 2010.000000 | 4692.000000 | 2065.000000 | 2010.000000 | 14.000000 | 3.000000 | 8.000000 | 4.000000 | 1418.000000 | 13.534474 |
λͺ¨λ 컬λΌμ numeric λ³μμ΄λ€. μ΄μμΉκ° μ‘΄μ¬νλ 컬λΌμ ~~ μ΄λ€. (μ€λ΅)
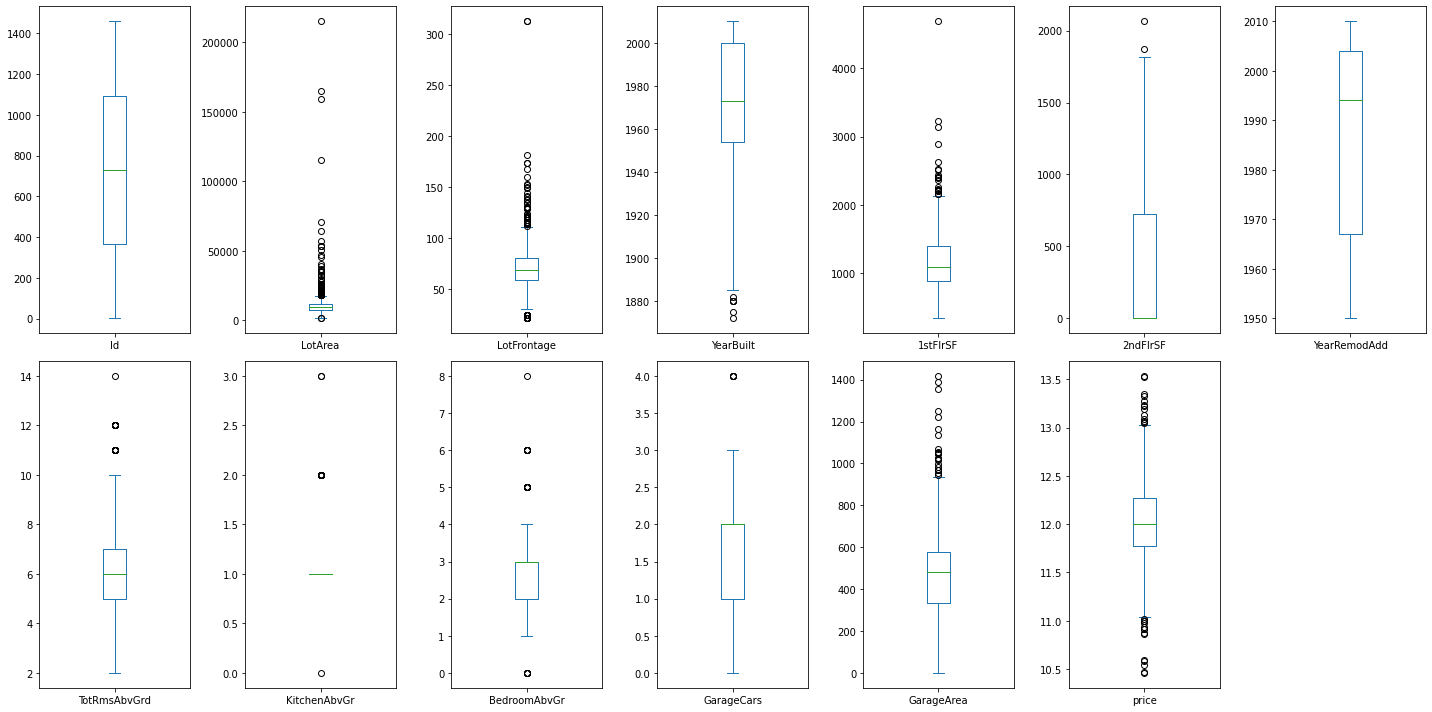
1-2λ²
Train,Valid,Test setμΌλ‘ λΆν λ° μκ°ν μ μ
df2 = df.copy()
#컬λΌμ μ«μκ° λ€μ΄κ°λ©΄ statsmodels ols λμμ μλ¬λ°μ
df2 = df2.rename(columns={'1stFlrSF':'first','2ndFlrSF':'second'})
#λ
λ λ°μ΄ν°μ κ²½μ° μ΅λλ
λ κΈ°μ€ λͺλ
μ μΈμ§ κ°μΌλ‘ λ체
df2['YearBuilt'] = abs(df2['YearBuilt'] - df2['YearBuilt'].max())
df2['YearRemodAdd'] = abs(df2['YearRemodAdd'] - df2['YearRemodAdd'].max())
X = df2.drop(columns=['Id','price','LotFrontage'])
y = df2['price']
from sklearn.model_selection import train_test_split
from sklearn.preprocessing import StandardScaler
X_train, X_test , y_train, y_test = train_test_split(X,y)
sc = StandardScaler()
sc.fit(X_train)
X_train_sc = sc.transform(X_train)
X_test_sc = sc.transform(X_test)
print('μ€μΌμΌλ§ μ μκ°ν')
X_train.plot(kind='box',subplots=True,layout=(2,len(df.columns)//2+1),figsize=(20,10))
plt.tight_layout()
plt.show()
print('μ€μΌμΌλ§ ν μκ°ν')
pd.DataFrame(X_train_sc,columns=X_train.columns).plot(kind='box',subplots=True,layout=(2,len(df.columns)//2+1),figsize=(20,10))
plt.tight_layout()
plt.show()
print('''
μ€λͺ
~~(μλ΅)) -> νκ· λΆμμ μ€μΌμΌλ§ νμ§ μλ κ²μ΄ r-squred κ°μ΄ λ λκ²λμ΄
''')
μ€μΌμΌλ§ μ μκ°ν
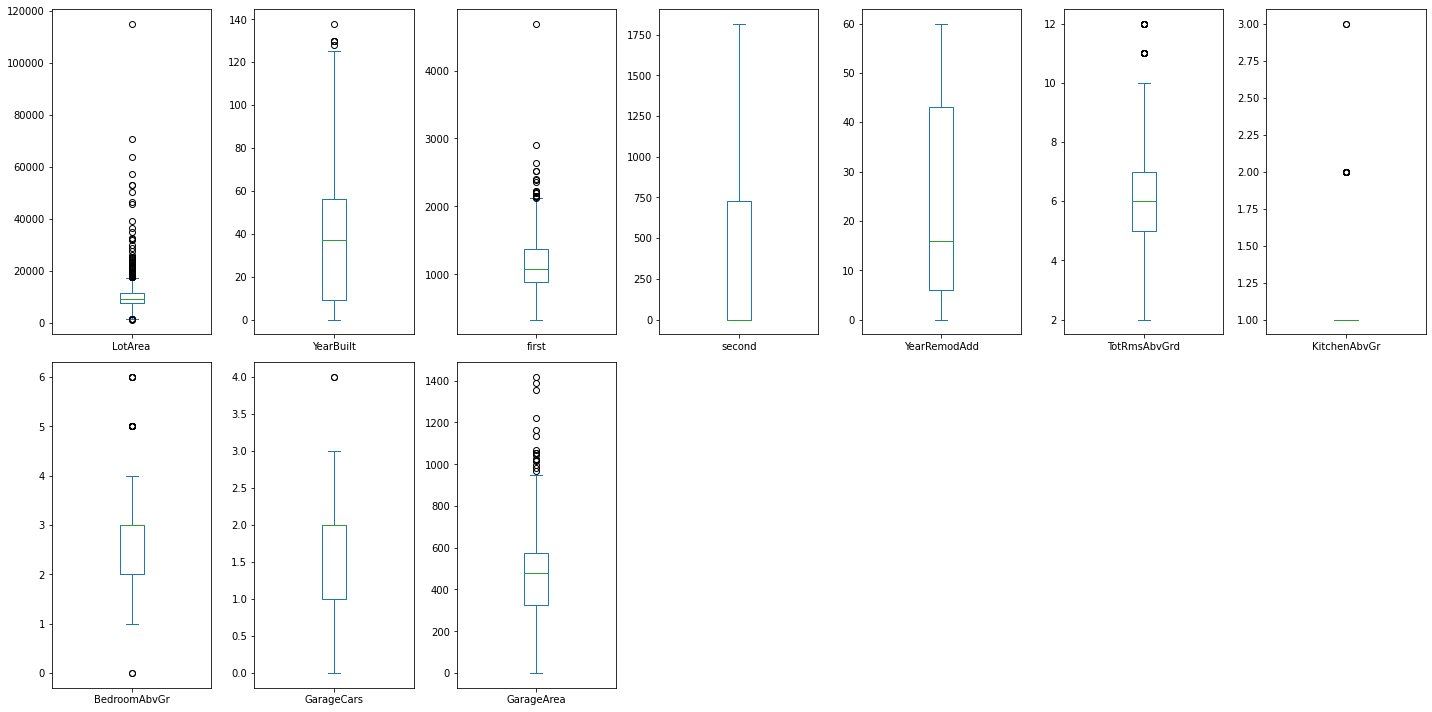
μ€μΌμΌλ§ ν μκ°ν
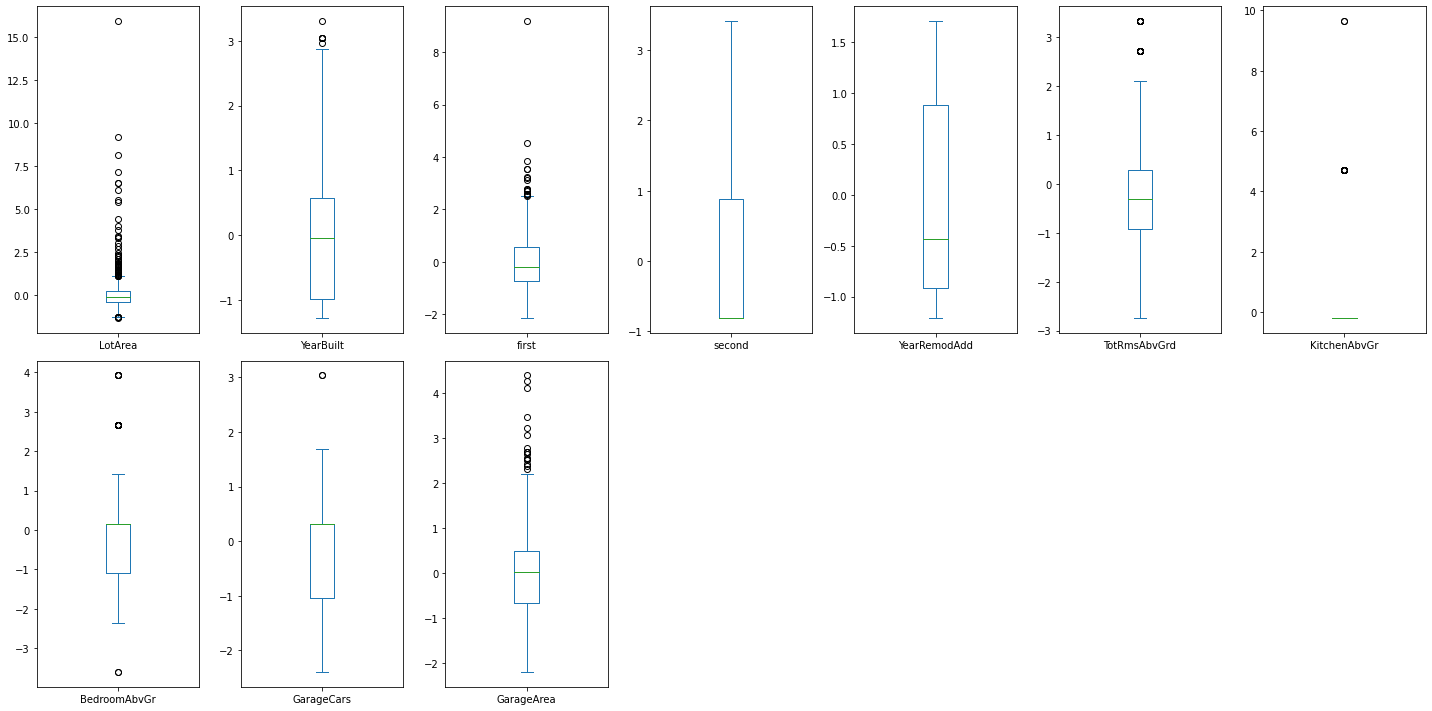
μ€λͺ
~~(μλ΅)) -> νκ· λΆμμ μ€μΌμΌλ§ νμ§ μλ κ²μ΄ r-squred κ°μ΄ λ λκ²λμ΄
1-3λ²
2μ°¨ κ΅νΈμμ©ν κΉμ§ κ³ λ €ν νκ·λΆμ μν λ° λ³μ μ ν κ³Όμ μ μ
from itertools import permutations
comb = list(permutations(X_train.columns, 3))
len(comb)
variables= '+ '.join(list(X_train.columns)) +'+'+ '+'.join([':'.join(list(y)) for y in comb])
# νμ΄μ¬μ νκ·λΆμμ μμ΄ λͺ¨λμ΄ λΆμΉμ² νκ±°κ°λ€μ γ
γ
γ
# μλμ 2μ°¨ κ΅νΈ μμ©μ λͺ¨λ ν¬ν¨ν 컬λΌμ€μμ κ°μμ κΈ°μ€μ λ§κ² λ³μ μ ννμλ©° λ κ² κ°μ΅λλ€.
# λͺ¨λ λ³μ ν¬ν¨μ λ¨μ λ€ννκ·λ³΄λ€λ r-squaredκ°μ΄ λκ² λμ΅λλ€
from statsmodels.formula.api import ols
#'+ '.join(list(X_train.columns))
res = ols(f'price ~ {variables}', data=pd.concat([X_train,y_train],axis=1)).fit()
res.summary()
Dep. Variable: | price | R-squared: | 0.886 |
---|---|---|---|
Model: | OLS | Adj. R-squared: | 0.871 |
Method: | Least Squares | F-statistic: | 57.61 |
Date: | Wed, 21 Dec 2022 | Prob (F-statistic): | 0.00 |
Time: | 01:16:19 | Log-Likelihood: | 643.13 |
No. Observations: | 1095 | AIC: | -1024. |
Df Residuals: | 964 | BIC: | -369.4 |
Df Model: | 130 | ||
Covariance Type: | nonrobust |
coef | std err | t | P>|t| | [0.025 | 0.975] | |
---|---|---|---|---|---|---|
Intercept | 11.1578 | 0.155 | 71.968 | 0.000 | 10.854 | 11.462 |
LotArea | 7.105e-06 | 5.78e-06 | 1.230 | 0.219 | -4.23e-06 | 1.84e-05 |
YearBuilt | -0.0031 | 0.001 | -3.560 | 0.000 | -0.005 | -0.001 |
first | 0.0007 | 0.000 | 5.077 | 0.000 | 0.000 | 0.001 |
second | 0.0004 | 0.000 | 3.585 | 0.000 | 0.000 | 0.001 |
YearRemodAdd | -0.0031 | 0.002 | -2.047 | 0.041 | -0.006 | -0.000 |
TotRmsAbvGrd | 0.0182 | 0.027 | 0.685 | 0.493 | -0.034 | 0.070 |
KitchenAbvGr | -0.1529 | 0.126 | -1.212 | 0.226 | -0.400 | 0.095 |
BedroomAbvGr | 0.0218 | 0.035 | 0.618 | 0.537 | -0.047 | 0.091 |
GarageCars | 0.1429 | 0.072 | 1.974 | 0.049 | 0.001 | 0.285 |
GarageArea | 7.146e-06 | 0.000 | 0.027 | 0.979 | -0.001 | 0.001 |
LotArea:YearBuilt:first | -4.009e-10 | 2.29e-10 | -1.752 | 0.080 | -8.5e-10 | 4.81e-11 |
LotArea:YearBuilt:second | 1.651e-10 | 2.52e-10 | 0.656 | 0.512 | -3.29e-10 | 6.59e-10 |
LotArea:YearBuilt:YearRemodAdd | -7.982e-09 | 3.35e-09 | -2.386 | 0.017 | -1.45e-08 | -1.42e-09 |
LotArea:YearBuilt:TotRmsAbvGrd | 1.608e-07 | 7.01e-08 | 2.295 | 0.022 | 2.33e-08 | 2.98e-07 |
LotArea:YearBuilt:KitchenAbvGr | 8.162e-07 | 2.99e-07 | 2.726 | 0.007 | 2.29e-07 | 1.4e-06 |
LotArea:YearBuilt:BedroomAbvGr | -3.453e-07 | 1.19e-07 | -2.909 | 0.004 | -5.78e-07 | -1.12e-07 |
LotArea:YearBuilt:GarageCars | -2.565e-07 | 1.84e-07 | -1.395 | 0.163 | -6.17e-07 | 1.04e-07 |
LotArea:YearBuilt:GarageArea | 3.271e-10 | 6.19e-10 | 0.528 | 0.597 | -8.88e-10 | 1.54e-09 |
LotArea:first:second | -1.82e-11 | 1.58e-11 | -1.156 | 0.248 | -4.91e-11 | 1.27e-11 |
LotArea:first:YearRemodAdd | -2.937e-10 | 3.13e-10 | -0.937 | 0.349 | -9.09e-10 | 3.21e-10 |
LotArea:first:TotRmsAbvGrd | -2.585e-09 | 2.48e-09 | -1.043 | 0.297 | -7.45e-09 | 2.28e-09 |
LotArea:first:KitchenAbvGr | -3.083e-08 | 2.22e-08 | -1.390 | 0.165 | -7.44e-08 | 1.27e-08 |
LotArea:first:BedroomAbvGr | 2.349e-08 | 8.21e-09 | 2.860 | 0.004 | 7.37e-09 | 3.96e-08 |
LotArea:first:GarageCars | -6.4e-09 | 9.49e-09 | -0.674 | 0.500 | -2.5e-08 | 1.22e-08 |
LotArea:first:GarageArea | 2.607e-11 | 2.45e-11 | 1.064 | 0.288 | -2.2e-11 | 7.41e-11 |
LotArea:second:YearRemodAdd | -5.768e-10 | 3.25e-10 | -1.775 | 0.076 | -1.21e-09 | 6.09e-11 |
LotArea:second:TotRmsAbvGrd | -6.54e-10 | 3.2e-09 | -0.205 | 0.838 | -6.93e-09 | 5.62e-09 |
LotArea:second:KitchenAbvGr | -4.56e-08 | 2.93e-08 | -1.555 | 0.120 | -1.03e-07 | 1.19e-08 |
LotArea:second:BedroomAbvGr | 3.259e-08 | 8.36e-09 | 3.901 | 0.000 | 1.62e-08 | 4.9e-08 |
LotArea:second:GarageCars | -2.455e-09 | 1.7e-08 | -0.145 | 0.885 | -3.58e-08 | 3.09e-08 |
LotArea:second:GarageArea | -2.17e-11 | 5.27e-11 | -0.412 | 0.681 | -1.25e-10 | 8.18e-11 |
LotArea:YearRemodAdd:TotRmsAbvGrd | -1.97e-08 | 9.19e-08 | -0.214 | 0.830 | -2e-07 | 1.61e-07 |
LotArea:YearRemodAdd:KitchenAbvGr | -5.82e-07 | 3.53e-07 | -1.651 | 0.099 | -1.27e-06 | 1.1e-07 |
LotArea:YearRemodAdd:BedroomAbvGr | 4.925e-07 | 1.4e-07 | 3.518 | 0.000 | 2.18e-07 | 7.67e-07 |
LotArea:YearRemodAdd:GarageCars | 3.294e-07 | 2.89e-07 | 1.138 | 0.255 | -2.38e-07 | 8.97e-07 |
LotArea:YearRemodAdd:GarageArea | -5.006e-10 | 9.76e-10 | -0.513 | 0.608 | -2.42e-09 | 1.41e-09 |
LotArea:TotRmsAbvGrd:KitchenAbvGr | 3.767e-06 | 6.03e-06 | 0.625 | 0.532 | -8.06e-06 | 1.56e-05 |
LotArea:TotRmsAbvGrd:BedroomAbvGr | -3.77e-06 | 1.7e-06 | -2.221 | 0.027 | -7.1e-06 | -4.39e-07 |
LotArea:TotRmsAbvGrd:GarageCars | 9.679e-06 | 4.96e-06 | 1.951 | 0.051 | -5.86e-08 | 1.94e-05 |
LotArea:TotRmsAbvGrd:GarageArea | -2.417e-08 | 1.46e-08 | -1.654 | 0.098 | -5.28e-08 | 4.5e-09 |
LotArea:KitchenAbvGr:BedroomAbvGr | -4.794e-07 | 9.78e-06 | -0.049 | 0.961 | -1.97e-05 | 1.87e-05 |
LotArea:KitchenAbvGr:GarageCars | 2.182e-05 | 2.02e-05 | 1.078 | 0.281 | -1.79e-05 | 6.15e-05 |
LotArea:KitchenAbvGr:GarageArea | -5.645e-08 | 7.28e-08 | -0.776 | 0.438 | -1.99e-07 | 8.63e-08 |
LotArea:BedroomAbvGr:GarageCars | -2.299e-05 | 6.54e-06 | -3.513 | 0.000 | -3.58e-05 | -1.01e-05 |
LotArea:BedroomAbvGr:GarageArea | 5.323e-08 | 2.25e-08 | 2.370 | 0.018 | 9.16e-09 | 9.73e-08 |
LotArea:GarageCars:GarageArea | 3.955e-09 | 5.75e-09 | 0.688 | 0.491 | -7.32e-09 | 1.52e-08 |
YearBuilt:first:second | 3.187e-09 | 3.26e-09 | 0.978 | 0.328 | -3.21e-09 | 9.58e-09 |
YearBuilt:first:YearRemodAdd | 4.164e-08 | 5.51e-08 | 0.755 | 0.450 | -6.66e-08 | 1.5e-07 |
YearBuilt:first:TotRmsAbvGrd | -3.609e-07 | 7.13e-07 | -0.506 | 0.613 | -1.76e-06 | 1.04e-06 |
YearBuilt:first:KitchenAbvGr | 5.498e-06 | 3.97e-06 | 1.386 | 0.166 | -2.28e-06 | 1.33e-05 |
YearBuilt:first:BedroomAbvGr | -6.066e-07 | 1.51e-06 | -0.401 | 0.689 | -3.58e-06 | 2.36e-06 |
YearBuilt:first:GarageCars | -7.33e-07 | 2.85e-06 | -0.257 | 0.797 | -6.33e-06 | 4.87e-06 |
YearBuilt:first:GarageArea | 1.572e-09 | 9.58e-09 | 0.164 | 0.870 | -1.72e-08 | 2.04e-08 |
YearBuilt:second:YearRemodAdd | -3.504e-08 | 5.05e-08 | -0.694 | 0.488 | -1.34e-07 | 6.41e-08 |
YearBuilt:second:TotRmsAbvGrd | -6.647e-07 | 6.43e-07 | -1.034 | 0.301 | -1.93e-06 | 5.97e-07 |
YearBuilt:second:KitchenAbvGr | 7.001e-06 | 3.79e-06 | 1.845 | 0.065 | -4.45e-07 | 1.44e-05 |
YearBuilt:second:BedroomAbvGr | -2.496e-07 | 1.17e-06 | -0.214 | 0.830 | -2.54e-06 | 2.04e-06 |
YearBuilt:second:GarageCars | -3.235e-06 | 2.23e-06 | -1.454 | 0.146 | -7.6e-06 | 1.13e-06 |
YearBuilt:second:GarageArea | 7.045e-10 | 8.18e-09 | 0.086 | 0.931 | -1.54e-08 | 1.68e-08 |
YearBuilt:YearRemodAdd:TotRmsAbvGrd | 1.83e-05 | 1.23e-05 | 1.483 | 0.138 | -5.91e-06 | 4.25e-05 |
YearBuilt:YearRemodAdd:KitchenAbvGr | -0.0001 | 3.46e-05 | -3.674 | 0.000 | -0.000 | -5.92e-05 |
YearBuilt:YearRemodAdd:BedroomAbvGr | 6.027e-06 | 1.94e-05 | 0.311 | 0.756 | -3.2e-05 | 4.41e-05 |
YearBuilt:YearRemodAdd:GarageCars | -4.087e-05 | 3.32e-05 | -1.231 | 0.219 | -0.000 | 2.43e-05 |
YearBuilt:YearRemodAdd:GarageArea | 1.983e-07 | 1.2e-07 | 1.647 | 0.100 | -3.8e-08 | 4.35e-07 |
YearBuilt:TotRmsAbvGrd:KitchenAbvGr | -0.0016 | 0.001 | -2.253 | 0.025 | -0.003 | -0.000 |
YearBuilt:TotRmsAbvGrd:BedroomAbvGr | 4.456e-05 | 0.000 | 0.192 | 0.848 | -0.000 | 0.000 |
YearBuilt:TotRmsAbvGrd:GarageCars | 0.0013 | 0.001 | 1.480 | 0.139 | -0.000 | 0.003 |
YearBuilt:TotRmsAbvGrd:GarageArea | -3.781e-06 | 2.94e-06 | -1.285 | 0.199 | -9.56e-06 | 1.99e-06 |
YearBuilt:KitchenAbvGr:BedroomAbvGr | 0.0011 | 0.001 | 0.865 | 0.387 | -0.001 | 0.004 |
YearBuilt:KitchenAbvGr:GarageCars | -0.0020 | 0.003 | -0.735 | 0.463 | -0.007 | 0.003 |
YearBuilt:KitchenAbvGr:GarageArea | -7.963e-06 | 9.58e-06 | -0.832 | 0.406 | -2.68e-05 | 1.08e-05 |
YearBuilt:BedroomAbvGr:GarageCars | -0.0003 | 0.001 | -0.228 | 0.820 | -0.003 | 0.002 |
YearBuilt:BedroomAbvGr:GarageArea | 7.721e-06 | 4.55e-06 | 1.695 | 0.090 | -1.22e-06 | 1.67e-05 |
YearBuilt:GarageCars:GarageArea | -8.145e-08 | 1.64e-06 | -0.050 | 0.961 | -3.31e-06 | 3.15e-06 |
first:second:YearRemodAdd | 1.258e-08 | 5.17e-09 | 2.433 | 0.015 | 2.43e-09 | 2.27e-08 |
first:second:TotRmsAbvGrd | 3.229e-08 | 4.27e-08 | 0.756 | 0.450 | -5.16e-08 | 1.16e-07 |
first:second:KitchenAbvGr | -6.114e-07 | 3.05e-07 | -2.004 | 0.045 | -1.21e-06 | -1.28e-08 |
first:second:BedroomAbvGr | -1.191e-07 | 9.06e-08 | -1.315 | 0.189 | -2.97e-07 | 5.87e-08 |
first:second:GarageCars | -2.4e-09 | 2.09e-07 | -0.011 | 0.991 | -4.13e-07 | 4.08e-07 |
first:second:GarageArea | 1.098e-09 | 6.6e-10 | 1.665 | 0.096 | -1.96e-10 | 2.39e-09 |
first:YearRemodAdd:TotRmsAbvGrd | -6.076e-07 | 1.07e-06 | -0.570 | 0.569 | -2.7e-06 | 1.48e-06 |
first:YearRemodAdd:KitchenAbvGr | 7.265e-06 | 6.33e-06 | 1.149 | 0.251 | -5.15e-06 | 1.97e-05 |
first:YearRemodAdd:BedroomAbvGr | -8.677e-07 | 2.14e-06 | -0.406 | 0.685 | -5.06e-06 | 3.33e-06 |
first:YearRemodAdd:GarageCars | -5.605e-06 | 4.85e-06 | -1.156 | 0.248 | -1.51e-05 | 3.91e-06 |
first:YearRemodAdd:GarageArea | 1.035e-08 | 1.66e-08 | 0.623 | 0.533 | -2.22e-08 | 4.29e-08 |
first:TotRmsAbvGrd:KitchenAbvGr | -4.385e-05 | 4.33e-05 | -1.013 | 0.311 | -0.000 | 4.11e-05 |
first:TotRmsAbvGrd:BedroomAbvGr | 1.291e-05 | 1.11e-05 | 1.161 | 0.246 | -8.91e-06 | 3.47e-05 |
first:TotRmsAbvGrd:GarageCars | -3.17e-05 | 3.7e-05 | -0.857 | 0.392 | -0.000 | 4.09e-05 |
first:TotRmsAbvGrd:GarageArea | 1.852e-07 | 1.18e-07 | 1.567 | 0.117 | -4.67e-08 | 4.17e-07 |
first:KitchenAbvGr:BedroomAbvGr | -8.223e-05 | 0.000 | -0.742 | 0.459 | -0.000 | 0.000 |
first:KitchenAbvGr:GarageCars | 5.371e-05 | 0.000 | 0.181 | 0.857 | -0.001 | 0.001 |
first:KitchenAbvGr:GarageArea | 1.37e-06 | 1.09e-06 | 1.253 | 0.210 | -7.75e-07 | 3.52e-06 |
first:BedroomAbvGr:GarageCars | 0.0002 | 0.000 | 1.731 | 0.084 | -2.48e-05 | 0.000 |
first:BedroomAbvGr:GarageArea | -1.124e-06 | 3.89e-07 | -2.890 | 0.004 | -1.89e-06 | -3.61e-07 |
first:GarageCars:GarageArea | -2.199e-07 | 1.33e-07 | -1.656 | 0.098 | -4.81e-07 | 4.07e-08 |
second:YearRemodAdd:TotRmsAbvGrd | -1.286e-06 | 1.02e-06 | -1.266 | 0.206 | -3.28e-06 | 7.07e-07 |
second:YearRemodAdd:KitchenAbvGr | -5.54e-06 | 5.93e-06 | -0.935 | 0.350 | -1.72e-05 | 6.09e-06 |
second:YearRemodAdd:BedroomAbvGr | 8.785e-07 | 1.87e-06 | 0.470 | 0.638 | -2.79e-06 | 4.55e-06 |
second:YearRemodAdd:GarageCars | 3.536e-06 | 4.02e-06 | 0.879 | 0.380 | -4.36e-06 | 1.14e-05 |
second:YearRemodAdd:GarageArea | -5.12e-09 | 1.45e-08 | -0.352 | 0.725 | -3.37e-08 | 2.34e-08 |
second:TotRmsAbvGrd:KitchenAbvGr | 0.0001 | 5.87e-05 | 2.102 | 0.036 | 8.2e-06 | 0.000 |
second:TotRmsAbvGrd:BedroomAbvGr | 1.177e-06 | 1.17e-05 | 0.101 | 0.920 | -2.18e-05 | 2.41e-05 |
second:TotRmsAbvGrd:GarageCars | -4.553e-05 | 4.18e-05 | -1.089 | 0.276 | -0.000 | 3.65e-05 |
second:TotRmsAbvGrd:GarageArea | -3.217e-08 | 1.4e-07 | -0.230 | 0.818 | -3.07e-07 | 2.42e-07 |
second:KitchenAbvGr:BedroomAbvGr | -0.0002 | 0.000 | -1.361 | 0.174 | -0.000 | 6.98e-05 |
second:KitchenAbvGr:GarageCars | 0.0003 | 0.000 | 0.948 | 0.343 | -0.000 | 0.001 |
second:KitchenAbvGr:GarageArea | 2.178e-07 | 1e-06 | 0.218 | 0.828 | -1.75e-06 | 2.18e-06 |
second:BedroomAbvGr:GarageCars | 4.715e-05 | 9.33e-05 | 0.506 | 0.613 | -0.000 | 0.000 |
second:BedroomAbvGr:GarageArea | -3.533e-07 | 3.15e-07 | -1.123 | 0.262 | -9.71e-07 | 2.64e-07 |
second:GarageCars:GarageArea | 3.037e-08 | 1.53e-07 | 0.199 | 0.842 | -2.69e-07 | 3.3e-07 |
YearRemodAdd:TotRmsAbvGrd:KitchenAbvGr | 0.0012 | 0.001 | 1.123 | 0.262 | -0.001 | 0.003 |
YearRemodAdd:TotRmsAbvGrd:BedroomAbvGr | -0.0003 | 0.000 | -0.883 | 0.377 | -0.001 | 0.000 |
YearRemodAdd:TotRmsAbvGrd:GarageCars | -0.0015 | 0.001 | -1.090 | 0.276 | -0.004 | 0.001 |
YearRemodAdd:TotRmsAbvGrd:GarageArea | 5.439e-06 | 4.78e-06 | 1.139 | 0.255 | -3.93e-06 | 1.48e-05 |
YearRemodAdd:KitchenAbvGr:BedroomAbvGr | -0.0007 | 0.002 | -0.393 | 0.695 | -0.004 | 0.003 |
YearRemodAdd:KitchenAbvGr:GarageCars | 0.0052 | 0.005 | 1.082 | 0.280 | -0.004 | 0.015 |
YearRemodAdd:KitchenAbvGr:GarageArea | -3.205e-06 | 1.65e-05 | -0.195 | 0.846 | -3.55e-05 | 2.91e-05 |
YearRemodAdd:BedroomAbvGr:GarageCars | 0.0034 | 0.002 | 1.891 | 0.059 | -0.000 | 0.007 |
YearRemodAdd:BedroomAbvGr:GarageArea | -1.564e-05 | 6.47e-06 | -2.418 | 0.016 | -2.83e-05 | -2.95e-06 |
YearRemodAdd:GarageCars:GarageArea | -4.013e-06 | 2.52e-06 | -1.593 | 0.111 | -8.96e-06 | 9.29e-07 |
TotRmsAbvGrd:KitchenAbvGr:BedroomAbvGr | 0.0172 | 0.014 | 1.227 | 0.220 | -0.010 | 0.045 |
TotRmsAbvGrd:KitchenAbvGr:GarageCars | -0.0201 | 0.062 | -0.323 | 0.747 | -0.142 | 0.102 |
TotRmsAbvGrd:KitchenAbvGr:GarageArea | -9.975e-05 | 0.000 | -0.387 | 0.699 | -0.001 | 0.000 |
TotRmsAbvGrd:BedroomAbvGr:GarageCars | -0.0065 | 0.023 | -0.280 | 0.780 | -0.052 | 0.039 |
TotRmsAbvGrd:BedroomAbvGr:GarageArea | 5.19e-05 | 8.26e-05 | 0.628 | 0.530 | -0.000 | 0.000 |
TotRmsAbvGrd:GarageCars:GarageArea | -1.463e-05 | 4.1e-05 | -0.357 | 0.721 | -9.51e-05 | 6.58e-05 |
KitchenAbvGr:BedroomAbvGr:GarageCars | -0.1440 | 0.093 | -1.553 | 0.121 | -0.326 | 0.038 |
KitchenAbvGr:BedroomAbvGr:GarageArea | 0.0004 | 0.000 | 1.121 | 0.263 | -0.000 | 0.001 |
KitchenAbvGr:GarageCars:GarageArea | -0.0002 | 0.000 | -1.475 | 0.141 | -0.001 | 7.82e-05 |
BedroomAbvGr:GarageCars:GarageArea | 0.0002 | 5.89e-05 | 3.060 | 0.002 | 6.46e-05 | 0.000 |
Omnibus: | 281.963 | Durbin-Watson: | 1.968 |
---|---|---|---|
Prob(Omnibus): | 0.000 | Jarque-Bera (JB): | 1549.607 |
Skew: | -1.071 | Prob(JB): | 0.00 |
Kurtosis: | 8.420 | Cond. No. | 8.76e+11 |
Notes:
[1] Standard Errors assume that the covariance matrix of the errors is correctly specified.
[2] The condition number is large, 8.76e+11. This might indicate that there are
strong multicollinearity or other numerical problems.
1-4λ²
λ²μ , μμλΈμ ν¬ν¨νμ¬ λͺ¨νμ μ ν©ν κΈ°κ³νμ΅ λͺ¨λΈ 3κ°μ§λ₯Ό μ μνλΌ
(νκ°μ§νλ MSE, MAPE, R2 λͺ¨λ νμΈν κ²)
# lasso , ridge , randomforest
import numpy as np
from sklearn.linear_model import Lasso
from sklearn.linear_model import Ridge
from sklearn.ensemble import RandomForestRegressor
from sklearn.metrics import mean_squared_error , r2_score
def MAPE(y_test, y_pred):
return np.mean(np.abs((y_test - y_pred) / y_test)) * 100
ls = Lasso()
rd = Ridge()
rf = RandomForestRegressor()
def modelpipe(model):
model.fit(X_train,y_train)
model_pred = model.predict(X_test)
mse = mean_squared_error(y_test,model_pred)
r2score = r2_score(y_test,model_pred)
mape = MAPE(y_test,model_pred)
metrics= [mse,r2score,mape]
return metrics
ls_result =modelpipe(ls)
rd_result =modelpipe(rd)
rf_result =modelpipe(rf)
result = pd.DataFrame([ls_result,rd_result,rf_result],columns = ['mse','r2','mape'],index=['lasso','ridge','randomForest'])
result
mse | r2 | mape | |
---|---|---|---|
lasso | 0.049039 | 0.697203 | 1.246075 |
ridge | 0.041681 | 0.742635 | 1.175805 |
randomForest | 0.037110 | 0.770859 | 1.094485 |
Attention
2λ²
λ°μ΄ν° μ€λͺ
: μ½λ‘λ19μ λν λλΌλ³ λ°μ΄ν°λ‘ λͺ¨λΈλ§ μ§ν
λ°μ΄ν° μΆμ² : https://www.kaggle.com/imdevskp/corona-virus-report μΌλΆ νμ²λ¦¬
data Url : https://raw.githubusercontent.com/Datamanim/datarepo/main/adp/p3/problem2.csv
location : μ§μλͺ
date : μΌμ
total_cases : λμ νμΈμ
total_deaths : λμ μ¬λ§μ
new_tests : κ²μ¬μ
population : μΈκ΅¬
new_vaccinations : λ°±μ μ μ’
μ
import pandas as pd
df =pd.read_csv('https://raw.githubusercontent.com/Datamanim/datarepo/main/adp/p3/problem2.csv')
df.head()
location | date | total_cases | total_deaths | new_tests | population | new_vaccinations | |
---|---|---|---|---|---|---|---|
0 | Afghanistan | 2020-02-24 | 5.0 | NaN | NaN | 39835428.0 | NaN |
1 | Afghanistan | 2020-02-25 | 5.0 | NaN | NaN | 39835428.0 | NaN |
2 | Afghanistan | 2020-02-26 | 5.0 | NaN | NaN | 39835428.0 | NaN |
3 | Afghanistan | 2020-02-27 | 5.0 | NaN | NaN | 39835428.0 | NaN |
4 | Afghanistan | 2020-02-28 | 5.0 | NaN | NaN | 39835428.0 | NaN |
2-1λ²
λ§μ§λ§ μΌμλ₯Ό κΈ°μ€μΌλ‘ μΈκ΅¬ λλΉ νμ§μ λΉμ¨μ΄ λμ μμ 5κ° κ΅κ°λ₯Ό ꡬνμ¬λΌ
μμ 5κ° κ΅κ°λ³λ‘ λμ νμ§μ, μΌμΌ νμ§μ, λμ μ¬λ§μ, μΌμΌ μ¬λ§μ, κ·Έλν, λ²λ‘λ₯Ό μ΄μ©ν΄μ κ°λ
μ± μκ² λ§λ€μ΄λΌ
df =pd.read_csv('https://raw.githubusercontent.com/Datamanim/datarepo/main/adp/p3/problem2.csv')
df['ratio'] = df['total_cases'] / df['population']
# μ 체 λ°μ΄ν°μ κ²°μΈ‘μΉ λ° μΌμΌ νμ§, μ¬λ§μ νμΈ
# 2021-11-30μλ new_tests , new_vaccinationsκ°μ΄ nan μ΄λ―λ‘ μ μΈ
# μΈκ΅¬μ 0μΈ μΌμ΄μ€ μ μΈ
import matplotlib.pyplot as plt
df = df.fillna(0)
df['date'] = pd.to_datetime(df['date'])
df = df[df.date != pd.to_datetime('2021-11-30')]
df = df[df.population !=0]
for location in df.location.unique():
lo = df[df.location == location]
df.loc[lo.index,'new_cases'] =lo.total_cases.diff().values
df.loc[lo.index[0], 'new_cases'] = lo['total_cases'].values[0]
df.loc[lo.index,'new_deaths'] =lo.total_deaths.diff().values
df.loc[lo.index[0], 'new_deaths'] = lo['total_deaths'].values[0]
df.loc[lo.index, 'total_vacciantions'] = lo['new_vaccinations'].cumsum().values
df.loc[lo.index, '7days_new_case'] = lo['new_tests'].rolling(7).sum().fillna(0).values
import seaborn as sns
import matplotlib.pyplot as plt
locations = df.groupby(['location']).tail(1).sort_values('ratio',ascending=False).location.head(5).values
target = df[df.location.isin(locations)].reset_index(drop=True)
for v in ['total_cases','new_cases','total_deaths','new_deaths']:
plt.figure(figsize = (15,5))
plt.title(v)
sns.lineplot(data=target,x= 'date',y=v,hue='location')
plt.show()
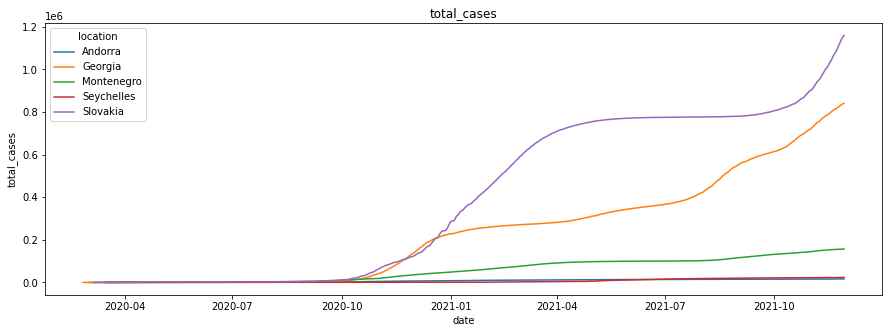
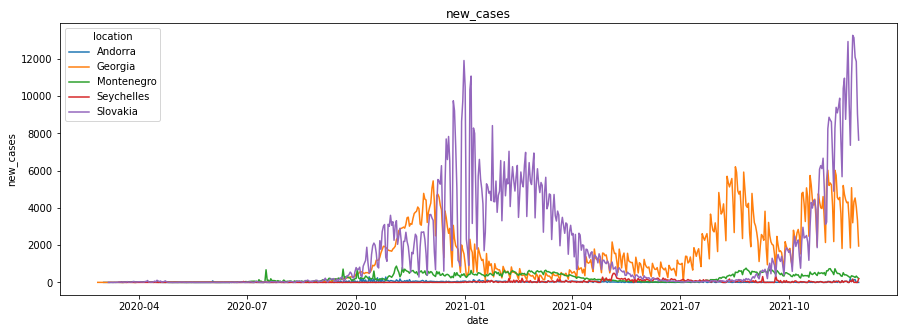
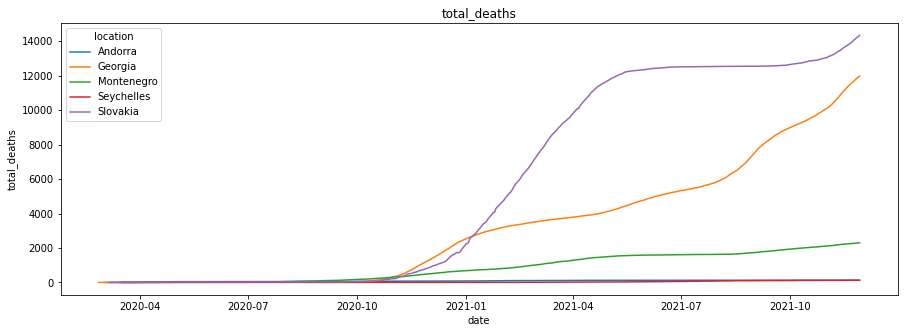
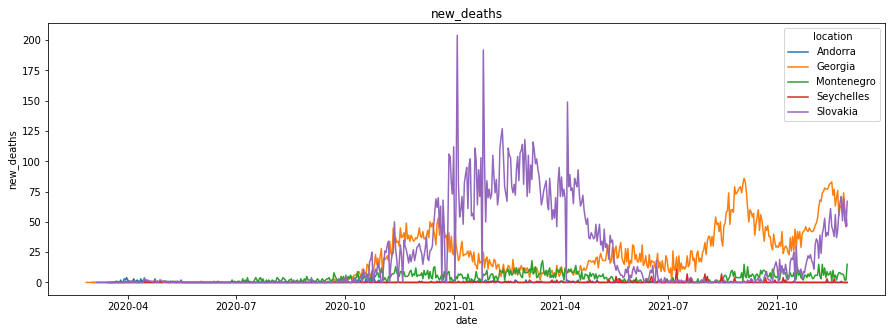
2-2λ²
μ½λ‘λ μνμ§μλ₯Ό μ§μ λ§λ€κ³ κ·Έ μνμ§μμ λν μ€λͺ μ μ κ³ μνμ§μκ° λμ κ΅κ°λ€ 10κ°λ₯Ό μ μ ν΄μ μκ°ν
# μνμ§μ = ( μ΅κ·ΌμΌμ£ΌμΌ λμ νμ§μ / μΈκ΅¬μ) + (μΌμΌ μ¬λ§μ / μΈκ΅¬μ) - (λμ λ°±μ μΈκ΅¬ / μΈκ΅¬μ) * 보μ μμ) * 보μ μμ
print('''
μ½λ‘λ μνμ§μλ μ½λ‘λλ‘ μΈν κ΅κ°μ μκΈ°μ λλ₯Ό νννλ€. μ½λ‘λ μ ν νΉμ±μ μ΅κ·Ό μΌμ£ΌμΌμ νμ§μ μ«μκ° κ·Έλ€μμ μΌμ£ΌμΌμ μν₯μ μ€λ€.
μΌμΌ μ¬λ§μμλ νμ¬ μ½λ‘λμ κ΅κ° λ΄μμμ μΉλͺ
μ¨μ νννλ€. μκΈ°μ λλ λμ λ°±μ μΈκ΅¬μ μν΄ κ°μ λ μ μλ€.
κ΅κ°κ°μ λΉκ΅λ₯Ό μν΄ κ° κ΅κ°μ μΈκ΅¬μλ‘ λλ μ£Όμ΄ κ°μ μ€μΌμΌλ§νκ³ , λ³μκ° λ³΄μ μμλ₯Ό ν΅ν΄ μ μνλ₯Ό μ λνλ€
''')
def ratio_index(x):
value = (x['7days_new_case'] / x['population'] + x['new_deaths'] / x['population'] - x['total_vacciantions'] / x['population']*0.001) *100
return value
df['ratio_index'] = df.apply(ratio_index,axis=1)
locations = df.groupby(['location']).tail(1).sort_values('ratio_index',ascending=False).location.head(10).values
target = df[df.location.isin(locations)].reset_index(drop=True)
for v in ['total_cases','new_cases','ratio_index']:
plt.figure(figsize = (15,5))
plt.title(v)
sns.lineplot(data=target,x= 'date',y=v,hue='location')
plt.show()
μ½λ‘λ μνμ§μλ μ½λ‘λλ‘ μΈν κ΅κ°μ μκΈ°μ λλ₯Ό νννλ€. μ½λ‘λ μ ν νΉμ±μ μ΅κ·Ό μΌμ£ΌμΌμ νμ§μ μ«μκ° κ·Έλ€μμ μΌμ£ΌμΌμ μν₯μ μ€λ€.
μΌμΌ μ¬λ§μμλ νμ¬ μ½λ‘λμ κ΅κ° λ΄μμμ μΉλͺ
μ¨μ νννλ€. μκΈ°μ λλ λμ λ°±μ μΈκ΅¬μ μν΄ κ°μ λ μ μλ€.
κ΅κ°κ°μ λΉκ΅λ₯Ό μν΄ κ° κ΅κ°μ μΈκ΅¬μλ‘ λλ μ£Όμ΄ κ°μ μ€μΌμΌλ§νκ³ , λ³μκ° λ³΄μ μμλ₯Ό ν΅ν΄ μ μνλ₯Ό μ λνλ€
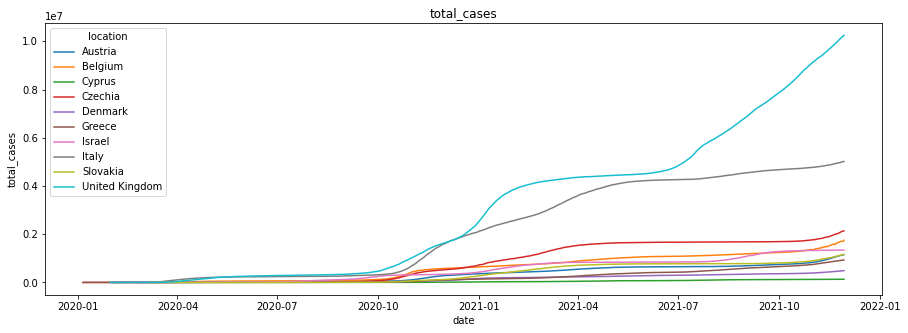
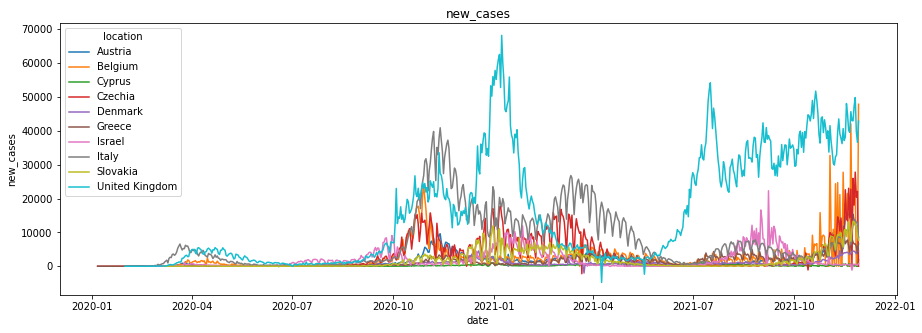
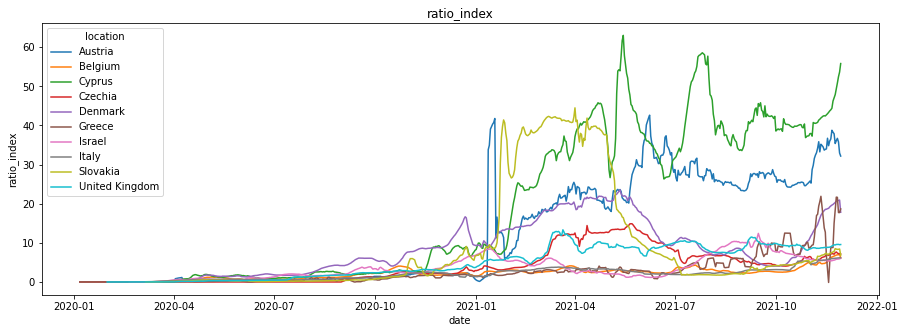
2-3λ²
νκ΅μ μ½λ‘λ μ κ· νμ§μ μμΈ‘ν΄λΌ(μ ν μκ³μ΄λͺ¨λΈ + λΉμ νμκ³μ΄ κ°κ° νκ°μ© λ§λ€μ΄λΌ)
μ νμκ³μ΄ - arma λΉμ ν μκ³μ΄ - arima
ko = df[df.location =='South Korea'].reset_index(drop=True)
ko.head()
location | date | total_cases | total_deaths | new_tests | population | new_vaccinations | ratio | new_cases | new_deaths | total_vacciantions | 7days_new_case | ratio_index | |
---|---|---|---|---|---|---|---|---|---|---|---|---|---|
0 | South Korea | 2020-01-21 | 0.0 | 0.0 | 0.0 | 51305184.0 | 0.0 | 0.000000e+00 | 0.0 | 0.0 | 0.0 | 0.0 | 0.0 |
1 | South Korea | 2020-01-22 | 1.0 | 0.0 | 5.0 | 51305184.0 | 0.0 | 1.949121e-08 | 1.0 | 0.0 | 0.0 | 0.0 | 0.0 |
2 | South Korea | 2020-01-23 | 1.0 | 0.0 | 0.0 | 51305184.0 | 0.0 | 1.949121e-08 | 0.0 | 0.0 | 0.0 | 0.0 | 0.0 |
3 | South Korea | 2020-01-24 | 2.0 | 0.0 | 0.0 | 51305184.0 | 0.0 | 3.898242e-08 | 1.0 | 0.0 | 0.0 | 0.0 | 0.0 |
4 | South Korea | 2020-01-25 | 2.0 | 0.0 | 0.0 | 51305184.0 | 0.0 | 3.898242e-08 | 0.0 | 0.0 | 0.0 | 0.0 | 0.0 |
# μ νλͺ¨λΈ - arma.
from statsmodels.tsa.ar_model import AutoReg
mod = AutoReg(ko.new_cases, 3, old_names=False)
res = mod.fit()
print(res.summary())
fig = res.plot_predict(1,700)
# λΉμ ν λͺ¨λΈ -arima μ¬μ©
from statsmodels.tsa.arima.model import ARIMA
model = ARIMA(ko.new_cases, order=(0,1,1))
model_fit = model.fit()
print(model_fit.summary())
forecast = model_fit.forecast(steps=24*7)
plt.figure(figsize=(10,5))
plt.plot(ko.new_cases)
plt.plot(forecast)
AutoReg Model Results
==============================================================================
Dep. Variable: new_cases No. Observations: 679
Model: AutoReg(3) Log Likelihood -4376.552
Method: Conditional MLE S.D. of innovations 156.844
Date: Wed, 21 Dec 2022 AIC 8763.103
Time: 01:16:32 BIC 8785.684
Sample: 3 HQIC 8771.846
679
================================================================================
coef std err z P>|z| [0.025 0.975]
--------------------------------------------------------------------------------
const 10.0652 7.966 1.264 0.206 -5.547 25.678
new_cases.L1 0.9978 0.037 27.163 0.000 0.926 1.070
new_cases.L2 -0.3117 0.052 -6.002 0.000 -0.413 -0.210
new_cases.L3 0.3080 0.038 8.196 0.000 0.234 0.382
Roots
=============================================================================
Real Imaginary Modulus Frequency
-----------------------------------------------------------------------------
AR.1 1.0045 -0.0000j 1.0045 -0.0000
AR.2 0.0037 -1.7978j 1.7978 -0.2497
AR.3 0.0037 +1.7978j 1.7978 0.2497
-----------------------------------------------------------------------------
SARIMAX Results
==============================================================================
Dep. Variable: new_cases No. Observations: 679
Model: ARIMA(0, 1, 1) Log Likelihood -4422.919
Date: Wed, 21 Dec 2022 AIC 8849.837
Time: 01:16:32 BIC 8858.876
Sample: 0 HQIC 8853.336
- 679
Covariance Type: opg
==============================================================================
coef std err z P>|z| [0.025 0.975]
------------------------------------------------------------------------------
ma.L1 0.0072 0.025 0.286 0.775 -0.042 0.057
sigma2 2.73e+04 486.188 56.156 0.000 2.63e+04 2.83e+04
===================================================================================
Ljung-Box (L1) (Q): 0.01 Jarque-Bera (JB): 8521.33
Prob(Q): 0.94 Prob(JB): 0.00
Heteroskedasticity (H): 21.35 Skew: 2.60
Prob(H) (two-sided): 0.00 Kurtosis: 19.57
===================================================================================
Warnings:
[1] Covariance matrix calculated using the outer product of gradients (complex-step).
[<matplotlib.lines.Line2D at 0x7f871a7941c0>]
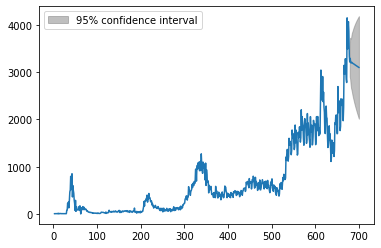
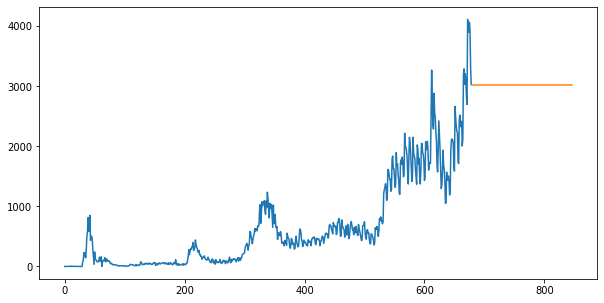
forecast = model_fit.forecast(steps=24*7)
plt.figure(figsize=(10,5))
plt.plot(ko.new_cases)
plt.plot(forecast)
[<matplotlib.lines.Line2D at 0x7f8702fd0370>]
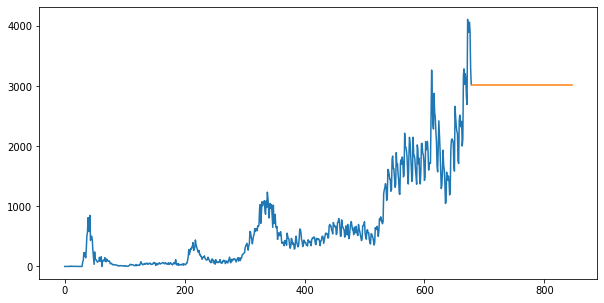
Attention
3λ²
μ€λ¬Έμ‘°μ¬ λ°μ΄ν°
λ°μ΄ν° μΆμ² : μ체 μ μ
data Url : https://raw.githubusercontent.com/Datamanim/datarepo/main/adp/p3/problem3.csv
λ°μ΄ν° μ€λͺ
: A ~ DκΉμ§μ κ·Έλ£Ήμκ² κ°κ° κ°μ μ€λ¬Έμ‘°μ¬λ₯Ό νμ¬ 1-1,1-2,1-3β¦5-1,5-4 μΈ μ€λ¬Έμ§λ₯Ό νΌ κ²μ΄λ€.
λ¬Ένμ μμλ³λ‘ λλμ΄ μκ³ , μμμ ν¬κ² 5κ°μ΄λ€(1~5)
κ° μμμ μΈλΆλ¬Ένμ 4κ°μ© μ‘΄μ¬νλ€ (1-1,1-2,1-3,1-4 ~) μ΄ λ μ€κ°μ λ°λ λ¬Ένμ΄ λ€μ΄κ° μλ€.
μλ₯Ό λ€μ΄ 1-1 λ¬Έμ κ° βλλ μκ°μ½μμ μ μ§ν¨λ€.βλΌλ λ¬Έμ λΌλ©΄ 1-3μ λ¬Έμ λ βλλ μκ°μ½μμ μ μ§ν€μ§ μλλ€.β λΌλ μλ¬Έμ λ‘ κ΅¬μ± λμ΄μλ€.
κ° μμμ 3λ²λ¬Ένμ 1λ²λ¬Ένμ μλ¬Έμ μ΄λ€. λͺ¨λ λ΅λ³μ 5μ μ²λμ΄λ€. λ¬Έμ λ₯Ό νκΈ°μ λͺ¨λ μλ¬Ένμ κ²½μ° μ μλ₯Ό λ³ν(6μ μ λΉΌμ) μμ
μ΄ νμνλ€
import pandas as pd
df =pd.read_csv('https://raw.githubusercontent.com/Datamanim/datarepo/main/adp/p3/problem3.csv')
df.head()
userid | group | Q1-1 | Q1-2 | Q1-3 | Q1-4 | Q2-1 | Q2-2 | Q2-3 | Q2-4 | ... | Q3-3 | Q3-4 | Q4-1 | Q4-2 | Q4-3 | Q4-4 | Q5-1 | Q5-2 | Q5-3 | Q5-4 | |
---|---|---|---|---|---|---|---|---|---|---|---|---|---|---|---|---|---|---|---|---|---|
0 | 0 | A | 5 | 2 | 1 | 2 | 4 | 5 | 3 | 3 | ... | 1 | 1 | 5 | 2 | 5 | 3 | 3 | 4 | 3 | 4 |
1 | 1 | A | 2 | 2 | 3 | 3 | 4 | 3 | 1 | 4 | ... | 2 | 3 | 4 | 3 | 5 | 3 | 1 | 2 | 1 | 1 |
2 | 2 | A | 1 | 3 | 4 | 4 | 2 | 1 | 4 | 4 | ... | 4 | 2 | 1 | 3 | 4 | 1 | 3 | 3 | 2 | 5 |
3 | 3 | A | 3 | 3 | 4 | 2 | 2 | 4 | 4 | 3 | ... | 2 | 3 | 3 | 4 | 2 | 4 | 1 | 1 | 3 | 2 |
4 | 4 | A | 3 | 1 | 2 | 3 | 4 | 3 | 4 | 1 | ... | 5 | 1 | 3 | 2 | 3 | 1 | 3 | 2 | 5 | 4 |
5 rows Γ 22 columns
3-1λ²
μλ¬Ένμ λ³ν ν ν κ° κ·Έλ£Ή(A~D)μ μμ(Q1~Q5)λ³ μλ΅μ νκ· , νμ€νΈμ°¨, μλ, 첨λλ₯Ό ꡬνλΌ. (κ° ν΅κ³λ λ³λ‘ 4x5 dataframe μμ±)
# μλ³ν
for num in range(1,6):
df[f'Q{num}-3'] =6 -df[f'Q{num}-3']
for num in range(1,6):
col_lst = ['group']
for col in range(1,5):
col_lst.append(f'Q{num}-{col}')
target = df[col_lst]
targetdf =target.set_index('group').unstack().to_frame().reset_index()[['group',0]].rename(columns ={0: f'Q{num}'})
display(targetdf.groupby('group').agg(['mean','std','skew',pd.DataFrame.kurt]))
Q1 | ||||
---|---|---|---|---|
mean | std | skew | kurt | |
group | ||||
A | 3.016 | 1.263860 | -0.077803 | -1.087887 |
B | 3.042 | 1.242489 | -0.126751 | -1.022905 |
C | 3.030 | 1.243642 | -0.050626 | -1.033246 |
D | 2.991 | 1.264325 | -0.069421 | -1.081406 |
Q2 | ||||
---|---|---|---|---|
mean | std | skew | kurt | |
group | ||||
A | 3.058 | 1.236999 | -0.129390 | -0.997133 |
B | 3.048 | 1.266215 | -0.111043 | -1.060834 |
C | 3.063 | 1.256427 | -0.122030 | -1.046603 |
D | 3.091 | 1.249913 | -0.166334 | -1.018150 |
Q3 | ||||
---|---|---|---|---|
mean | std | skew | kurt | |
group | ||||
A | 2.992 | 1.268679 | -0.061600 | -1.098330 |
B | 3.050 | 1.238965 | -0.117158 | -1.035672 |
C | 3.023 | 1.248210 | -0.102330 | -0.988577 |
D | 3.034 | 1.255556 | -0.128043 | -1.043094 |
Q4 | ||||
---|---|---|---|---|
mean | std | skew | kurt | |
group | ||||
A | 3.043 | 1.255678 | -0.090314 | -1.028166 |
B | 3.041 | 1.240507 | -0.071541 | -1.014676 |
C | 3.014 | 1.283531 | -0.074531 | -1.100094 |
D | 3.080 | 1.268546 | -0.144620 | -1.006126 |
Q5 | ||||
---|---|---|---|---|
mean | std | skew | kurt | |
group | ||||
A | 3.088 | 1.256119 | -0.102638 | -1.053632 |
B | 2.983 | 1.272136 | -0.055805 | -1.080934 |
C | 2.987 | 1.260325 | -0.068696 | -1.071557 |
D | 2.989 | 1.250777 | -0.065315 | -1.055332 |
3-2λ²
κ·Έλ£Ήλ³λ‘ Q1-1λ¬Ένμ μ°¨μ΄κ° μ‘΄μ¬νλμ§ anovaλΆμμ μννλΌ
from scipy.stats import shapiro
a = df[df.group =='A']['Q1-1']
b = df[df.group =='B']['Q1-1']
c = df[df.group =='C']['Q1-1']
d = df[df.group =='D']['Q1-1']
print('a p-value',shapiro(a)[1])
print('b p-value',shapiro(b)[1])
print('c p-value',shapiro(c)[1])
print('d p-value',shapiro(d)[1])
from scipy.stats import levene
# λ±λΆμ° λ§μ‘±νλ€
print(levene(a,b,c,d))
print()
# μ κ·μ±μ λ§μ‘±νμ§ μκΈ° λλ¬Έμ kruskal-wallis H testλ₯Ό ν΅ν΄ λΆμ° λΆμ μ§ν
from scipy.stats import kruskal
kruskal(a,b,c,d)
# 4κ°μ κ·Έλ£Ήμ ν΅κ³μ μΌλ‘ μ μν μ°¨μ΄κ° μλ€
a p-value 4.089666539447423e-12
b p-value 1.2895768654319628e-11
c p-value 1.4126045819184974e-11
d p-value 4.2081052184506085e-12
LeveneResult(statistic=0.24718103455049822, pvalue=0.8633690011210747)
KruskalResult(statistic=4.567127187870985, pvalue=0.20638028098088249)
3-3λ²
νμμ μμΈλΆμμ μννκ³ κ²°κ³Όλ₯Ό μκ°ν νλΌ
ana = df.drop(columns = ['userid','group'])
#μ€μ adp ν¨ν€μ§λ¦¬μ€νΈμλ μ‘΄μ¬ν¨
#!pip install factor-analyzer
from factor_analyzer.factor_analyzer import calculate_bartlett_sphericity
chi_square_value,p_value=calculate_bartlett_sphericity(ana)
chi_square_value, p_value
# μμΈμ± νκ° κ²°κ³Ό μμΈμ± νκ°μ μ ν©ν p-value( <0.05)λ₯Ό νμΈ
from factor_analyzer import FactorAnalyzer
from factor_analyzer.factor_analyzer import calculate_kmo
kmo_all,kmo_model=calculate_kmo(ana)
kmo_model
# kmo κ²°κ³Ό 0.6 μ΄νλ λΆμ ν©νλ€ λ³Έλ€
fa = FactorAnalyzer(n_factors=25,rotation=None)
fa.fit(ana)
#Eigenκ° μ²΄ν¬
ev, v = fa.get_eigenvalues()
plt.scatter(range(1,ana.shape[1]+1),ev)
plt.plot(range(1,ana.shape[1]+1),ev)
plt.title('Scree Plot')
plt.xlabel('Factors')
plt.ylabel('Eigenvalue')
plt.grid()
plt.show()
#eigenvalueκ° 1μ΄ λλμ§μ μΈ 10κ°μ μμΈμ΄ μ νμ μ ν©ν μ«μλ‘ νμΈ
fa = FactorAnalyzer(n_factors=10, rotation="varimax") #ml : μ΅λμ°λ λ°©λ²
fa.fit(ana)
efa_result= pd.DataFrame(fa.loadings_, index=ana.columns)
plt.figure(figsize=(6,10))
sns.heatmap(efa_result, cmap="Blues", annot=True, fmt='.2f')
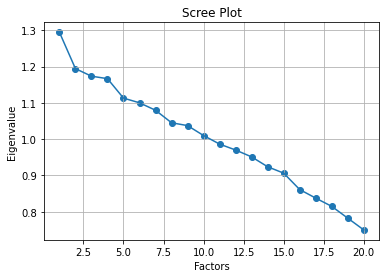
<AxesSubplot:>
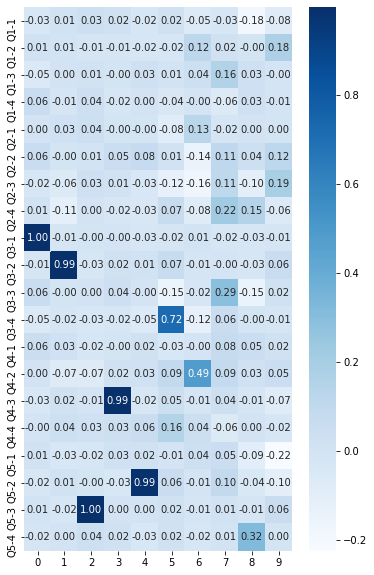